Recommendation engines can be implemented for a range of purposes and use cases, but they’re perhaps best known (and at their most effective) when used to serve product recommendations to ecommerce consumers.
With 91% of consumers saying they prefer to shop with ecommerce stores that provide relevant offers and recommendations, providing accurate suggestions to users is now more of a necessity than a nice-to-have.
In this article, we’ll take a look at how product recommendations work, and how you can easily deploy one on your ecommerce store.
What is an ecommerce recommendation engine?
An ecommerce recommendation engine is a tool used to provide product recommendations to consumers on an ecommerce website. The recommendations can be surfaced across various areas of the website, including category pages, product pages and checkout or basket pages.
Here’s an example of a product recommendation in action, from ecommerce cosmetics retailer, Lush:
The purpose of product recommendations is to highlight relevant products that are likely to be of interest to users and which they are likely to purchase. This has several benefits for ecommerce organisations:
Improve conversion rates
Well-placed and relevant product recommendations can help ecommerce sites to improve their conversion rates. Because you’re showing users the products they’re more likely to have an interest in, you increase their propensity to purchase and therefore will likely see an upturn in conversions. According to Google, recommendations can improve conversion rate by up to 40%.
Increase basket size
As well as simply getting users to buy, recommendation engines can make them buy more. By showing complementary products at product page or checkout stages, you can highlight additional purchases that may be of interest, based on that user’s previous behaviour or what they’ve already viewed or added to their basket. As they’ve already already shown purchase intent, you can effectively upsell and cross-sell to these customers to increase their basket size.
Increase revenue and average order value
Given that product recommendations can encourage customers to buy — and buy more — it makes sense that this results in higher revenues and average order values for ecommerce sites. As users add more products to their basket, they spend more.
Product recommendations can also be used to increase revenues through directing users to bigger/more valuable versions of the same product, or perhaps additional products sold in a bundle with an attractive ‘bulk buy’ discount.
Google’s data shows that revenues can increase by up to 50% through targeted recommendations.
How do you implement a recommendation engine?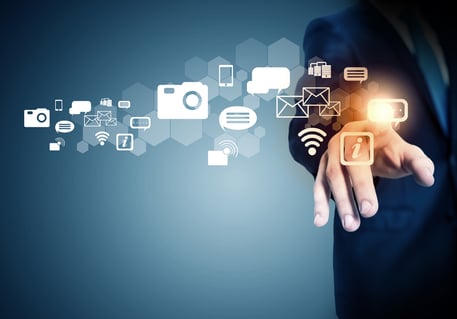
To deploy the recommendations to your site, you have two options when it comes to the engine powering the feature: build one, or use a ready-made solution. Whichever option you choose, you’ll need to understand the importance of data organisation and storage, and which filtering systems can help deliver the best product recommendations.
The quality of a recommendation engine’s output depends on the set up and implementation, as well as the quality of the data fed into the engine.
The implementation needs to consider and include:
Data ingestion -
The data you’ll use to power the recommendations is imperative if you want to provide accurate and relevant recommendations to your users. This means that it’s important for the engine to be able to ingest data from your chosen sources, and to be able to use that data effectively to deliver the right results.
Filtering -
The filtering of the data is just as important, as this will ultimately decide which products are recommended to your website users. You need to define the goals of the recommendations and to define the criteria behind the logic of what’s served to the end user.
This is done through filtering the data in one of two ways:
1. Content-based filtering
Content-based filtering works off an individual user’s activity and preferences. Content is first labelled with attributes or keywords, and the recommendation engine then captures the attributes of the content a user interacts with (in this case the products they view and/or purchase). Other products using the same tags are then identified by the engine and shown to the user. The accuracy of the recommendations largely depends on the quality of the tagging on the content and the amount of information captured about users — so the onus remains on merchandising and ecommerce teams to ensure the product catalogue is accurately tagged.
2. Collaborative filtering
Collaborative filtering works slightly differently, and uses data from many users to identify behavioural patterns. For example, the engine recognises people who show interest in certain products or display certain behavioural attributes, and recommend those products to other people who behave similarly.
This type of filtering is often used in product recommendation engines; Amazon’s “People who bought this also bought…” feature is generated through collaborative filtering. Collaborative recommendation engines are considered to offer more relevant recommendations, while they can help users discover relevant products more easily.
Of course, using a ready-to-go recommendation engine is beneficial because it prevents the need for lengthy and costly development work, and can be up and running relatively quickly. Ancoris CMOLab Recommendation Engine provides a plug-and-play solution that connects with your existing data sources and ecommerce platform to provide intelligent recommendations to your customers.
Ancoris CMOLab: A powerful product recommendation engine for your ecommerce store
Ancoris CMOLab Recommendation Engine is powered by Google Cloud, harnessing the power of Google’s machine learning capabilities to enable you to deliver meaningful product recommendations to your customers.
Developed to provide highly accurate consumer recommendations, leveraging the very latest in Google Cloud AI, and data analytics technology, Ancoris CMOLab easily connects to your existing eCommerce, ERP and content management systems. Once connected, recommendations are computed on a daily basis, and served to consumers via simple integration with your existing customer facing platforms.
Ready to delight your customers, improve your conversion rates and increase your revenues with powerful, accurate and relevant product recommendations?
Get in touch today and let’s discuss how Ancoris CMOLab Recommendation Engine can help.